Key insights from the symposium on AI and Academic Publishing
On 7 November 2024, the UBL and Elsevier jointly organised a symposium on AI and academic publishing.
During the last few decades, it has become clear that artificial intelligence (AI) can have far-reaching consequences for all actors in the realm of scholarly communication, including authors, editors, researchers, funders, and readers. On 7 November 2024, the UBL and Elsevier jointly organised a symposium on AI and academic publishing to foster and broaden the conversation about the various ways in which AI can augment or automate aspects of academic publishing.
Michael Cook opened the symposium with a principled reflection on why we write and why we publish. He argued that, in the context of academic publishing, AI can help us to curate and disclose the broader historical context of scientific discoveries. When we want to understand the nature and the impact of scholarly contributions made by researchers, academic publications alone may not be sufficient. We also need to consider sources such as marginal notes, correspondence and research annotations. In the digital age, such information about the social and cultural context of ideas and inventions can also be found on YouTube and on social media. AI can help us to draw connections between these various online sources. Cook compared scholarly communication to an overgrown garden, and suggested that AI may be used to maintain and tend to this garden. You can watch the full lecture on Leiden University's video portal.
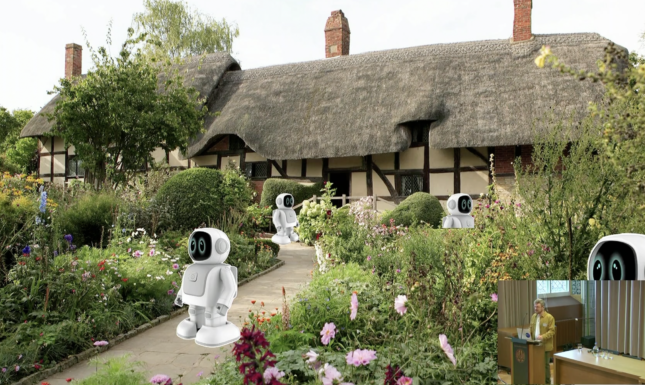
Catholijn Jonker and Niklas Höpner spoke about hybrid intelligence, the combination of human and machine intelligence. When humans collaborate with machines, this can augment human intelligence in various ways. AI-based research assistants form one example of such environments in which human intelligence and machine intelligence meet. Examples include Consensus, Sakana, and Jenni.ai. Tools such as these may be used to write papers, to generate hypotheses, and to analyse data. Hopner indicated that, while such tools can be helpful in some situations, they still have numerous shortcomings. They may implement ideas incorrectly, or they can miss key references to other works. We still lack reliable methods to evaluate the performance of such research assistants. It was stressed that therefore a hybrid approach is still necessary. Artificial research assistants can result in sound, relevant and ethical research only if human researchers can monitor and guide their methods and their outputs. You can watch the full lecture on Leiden University's video portal.
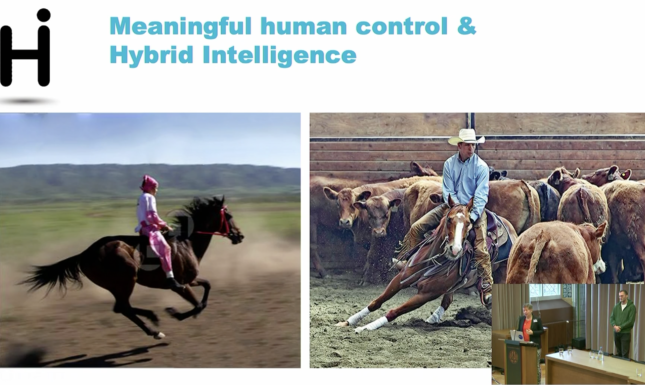
Peter van der Putten addressed the question whether AI can be viewed as a metaphor for the whole scientific endeavour. By studying AI, we may develop a better understanding of the nature of intelligence in a broader sense, and, ultimately, of ourselves as well. A problem, however, is that AI typically shows us a representation of reality rather than reality itself, analogous to the way in which the prisoners in Plato’s cave only looked at shadows of phenomena. The full lecture can be watched here.
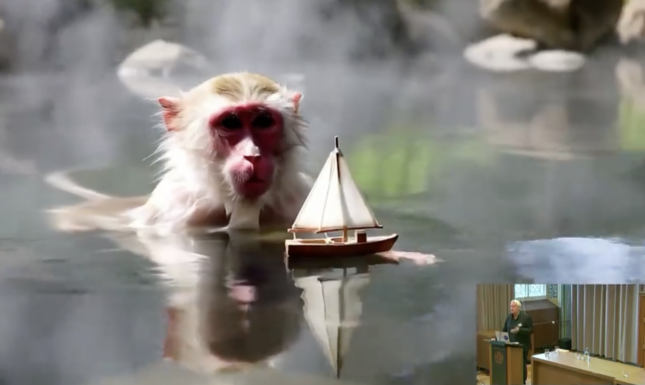
In his talk entitled “AI in Science Means AI in Publishing”, Paul Groth discussed applications of AI within a broad range of disciplines. As was also shown in the report Artificial Intelligence in Science, which was commissioned by the EU, there is a considerable uptake of AI-based methods in fields across the full academic spectrum, including the physical sciences, the life sciences and the social sciences. Groth explained that AI has been used to discover new antibiotics, to develop new materials, to improve weather forecasts and to analyse nuclear fusions. The talk ended with a reflection on the implications for academic publishing. It is clear, firstly, that, next to papers, researchers currently produce many different forms of output, such as videos, models, social media posts and simulations. These multimodal resources need to be curated well, as they can be valuable for AI systems. It is also important to ensure the quality of these resources and to describe their provenance diligently. You can watch the full talk on the Leiden University video portal.
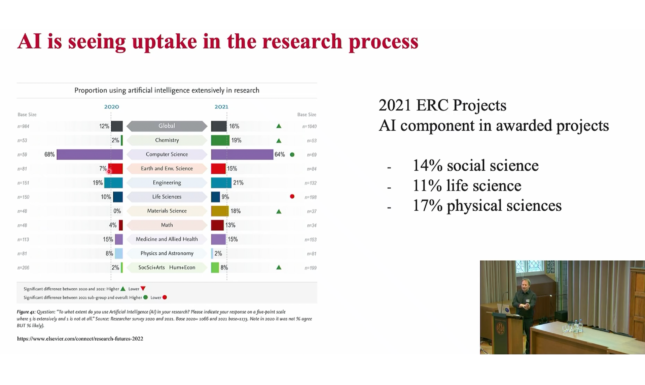
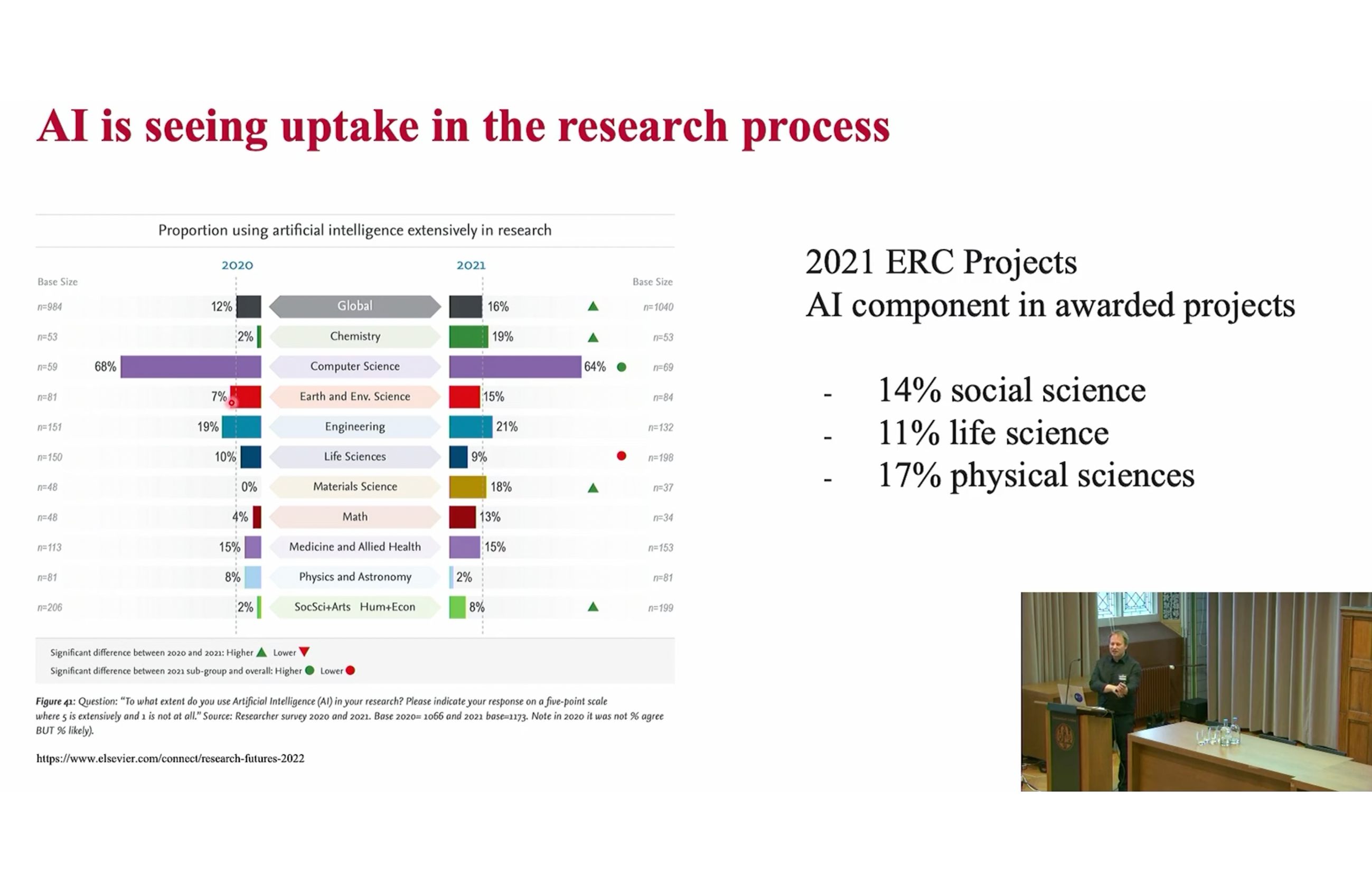
Anita de Waard, VP of Research Collaborations at Elsevier, noted that generative AI may affect research integrity. AI systems can easily be used to fabricate papers and data, and there have been many cases in which such fake publications have passed though the peer review process. RELX has formulated a set of principles to promote the responsible use of AI. Among other things, these principles emphasise the importance of considering the real-world impact of solutions on people, and of preventing the creation or reinforcement of unfair bias. It is pivotal, furthermore, to be able to explain how AI-based solutions actually work, and to create accountability via human oversight. Elsevier has taken a number of measures to reduce biases in AI systems and to record the provenance of data. Authors are allowed to use generative AI to edit their texts, and to polish up the style, but they continue to bear the responsibility for their text. Generative AI should not be listed as a co-author. The full presentation is available through the Leiden University video portal.
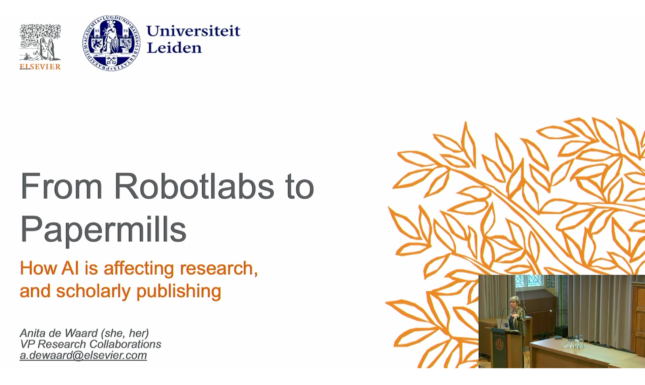
The final panel discussion was moderated by Kathleen Gregory. The discussion focused on the implications of AI for the core values that characterise academic research. One of these values is transparency. In the context of AI research, transparency can be enhanced by documenting the data and parameters used in the training process. One issue is that the inner workings of AI models are often difficult to explain, and this ‘epistemic opacity’ may undermine transparency. Another value, linked closely to transparency, is reproducibility. When all the data, software and models used in a study are curated, this enables others the trace all the steps that were followed in a research project. Training a new AI model usually demands enormous amounts of time and money, however. As a result, the results obtained via such models cannot easily be replicated by researchers working with smaller budgets. At the same time, it is also clear that the costs of inferencing, and of using pre-trained models have decreased considerably. This, in turn, helps to decrease the inequalities between institutions.
The symposium has shown that AI can have important implications for some of the central goals and some of the core values underpinning academic publishing. AI can help to improve accessibility, reproducibility and equity, but it also poses considerable challenges to the integrity and the reliability of academic knowledge.
More information
The slides of the speakers at this symposium can all be found on Zenodo (https://doi.org/10.5281/zenodo.14362027).
Abstracts and brief bibliographies of the speakers can be found on the UBL website.
The video recordings can be found on the Leiden University video portal:
- Welcome
- Keynote by Michael Cook
- Presentation by Cathlijn Jonker and Niklas Höpfer
- Presentation by Peter van der Putten
- Presentation by Paul Groth
- Presentation by Anita de Waard
- Panel discussion
See also the blog by Alastair Dunning (TU Delft)